A DIY Guide to Differential Gene Expression Analysis
Unraveling Patterns in Gene Expression With Differential Expression Analysis
Understanding the language of genes is akin to deciphering the secrets of life. One powerful tool in this decoding process is differential expression analysis — a method that unveils how genes are being “turned on” and “turned off” in response to different biological conditions.
In this edition of Decoding Biology, I’ll teach you what differential expression analysis is, when and why it’s used, and how you can perform it yourself. As always, if you enjoyed this newsletter, I’d be grateful if you’d consider tapping the “heart” 💙 in the header above. It helps me understand which pieces you like most and supports this newsletter’s growth. Thank you!
🧫 What Is Differential Gene Expression?
Gene expression is the process by which information encoded in a gene is used to create a functional gene product, typically a protein or RNA molecule. The first step of gene expression is transcription, which is when the DNA sequence coding for a gene is transcribed into messenger RNA (mRNA) by the enzyme RNA polymerase.
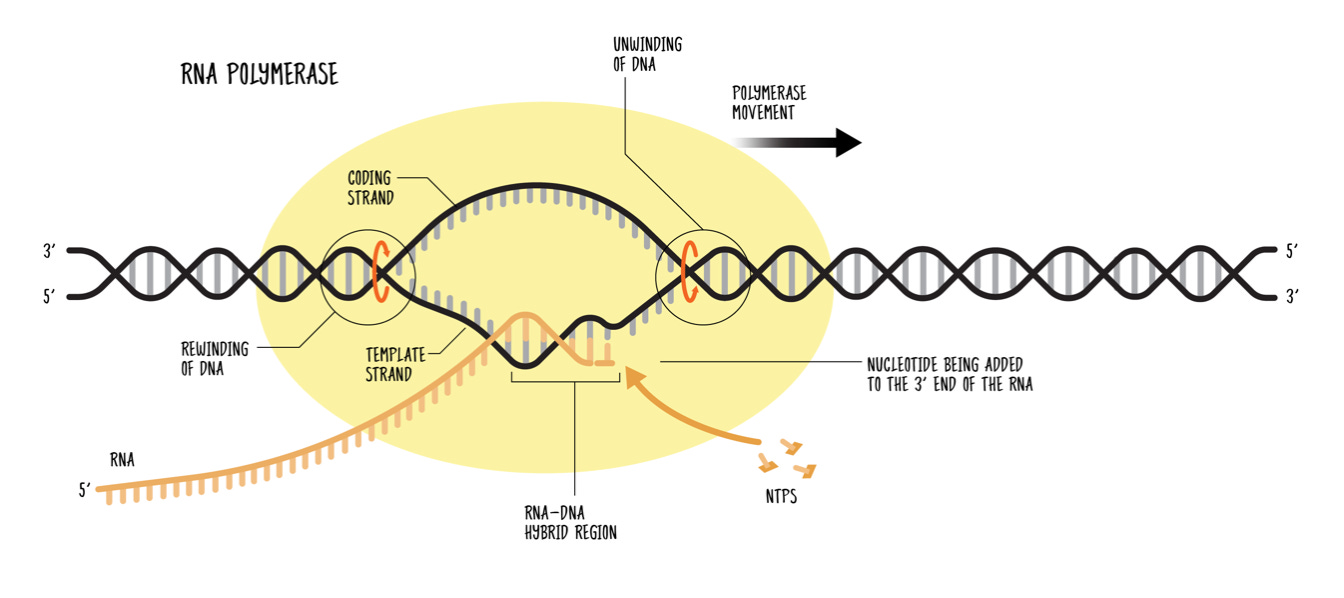
The mRNA is then translated into a function protein with the help of ribosomes and transfer RNA (tRNA), as shown in the image below. Gene expression is a tightly regulated process that allows cells to respond to environmental cues, perform specific functions, and adapt to changing conditions. Not all genes are expressed at all times, and the expression level can vary between different cell types, tissues, and developmental stages. In other words, genes can be differentially expressed, which refers to the ability of genes to be "turned on" or "turned off" in response to specific factors or changes in the cellular environment.
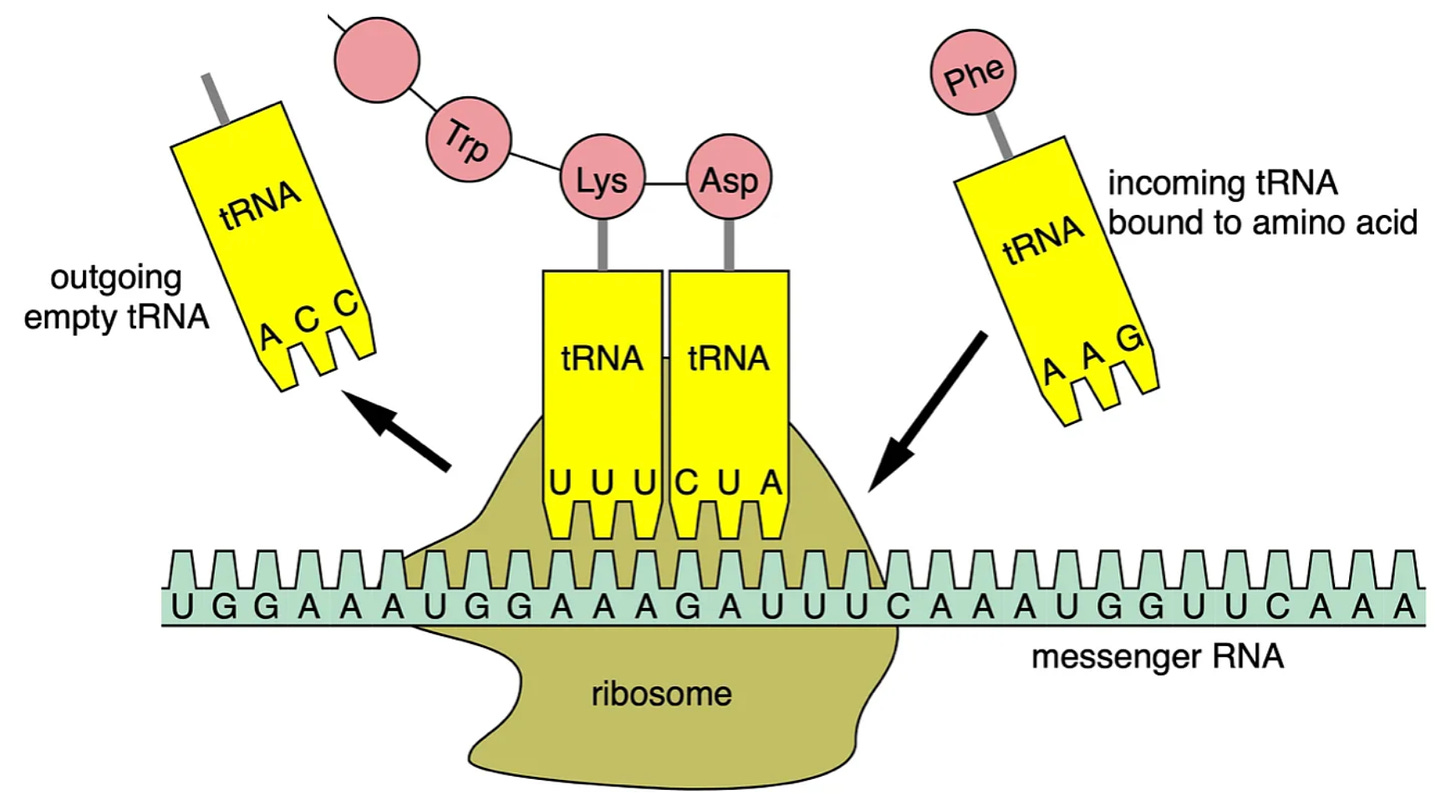
Differential expression analysis is a fundamental technique in bioinformatics used to identify differentially expressed genes between two or more biological conditions, such as healthy and diseased tissues, or before and after a treatment. To perform differential expression analysis, we must perform statistical analysis to discover quantitative changes in expression levels between experimental groups. For example, we use statistical testing to decide whether, for a given gene, an observed difference in read counts is significant, that is, whether it is greater than what would be expected just due to natural random variation.
🧫 How Do We Know Genes Are Differentially Expressed?
Differential expression analysis aims to understand how gene expression levels change under different conditions, providing insights into the molecular mechanisms underlying biological processes.
Before performing differential expression analysis, we need to quantify gene expression levels. This can be done with RNA sequencing (RNA-seq) and microarrays, which are used to identify genes being actively transcribed. RNA-Seq, a cutting-edge technique, reads the entire transcriptome, providing a nuanced and dynamic understanding of gene activity. In contrast, microarrays, akin to genetic snapshots, capture a snapshot of gene expression levels at a given moment.
After obtaining gene expression data, we can assess differential expression by comparing the expression of genes under different experimental conditions. For example, researchers might compare gene expression in healthy and diseased tissues or in the presence and absence of a specific treatment, or they may investigate expression under different environmental conditions, as demonstrated in the figure below reproduced from a study titled, Role and mechanism of the AMPK pathway in waterborne Zn exposure influencing the hepatic energy metabolism of Synechogobius hasta:
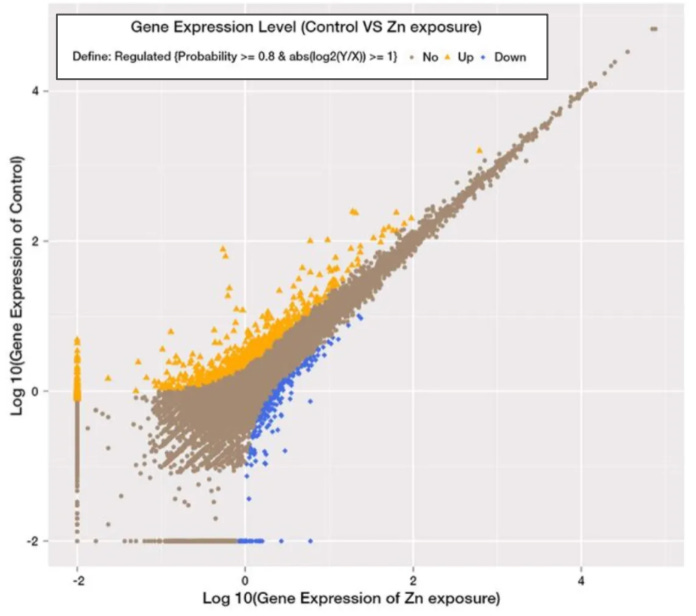
A gene is considered upregulated if its expression increases by a statistically significant degree in a particular condition and downregulated if its expression decreases by a statistically significant degree. In the image above, genes that are upregulated in the control group as compared to the zinc-exposed group are indicated in orange, whereas genes that are down-regulated in the control group as compared to the zinc-exposed group are indicated in blue. The genes indicated in brown weren't differentially expressed between the two groups. In the next section, we'll explore how we can determine this ourselves, using the raw data from a study titled, Amiloride, An Old Diuretic Drug, Is a Potential Therapeutic Agent for Multiple Myeloma.
🧫 DIY Differential Gene Expression Analysis
In this section, I’m going to show you how to perform differential gene expression analysis yourself, using data from a study titled, Amiloride, An Old Diuretic Drug, Is a Potential Therapeutic Agent for Multiple Myeloma1.
The data from this study has been made available through the Gene Expression Omnibus, which is a public database containing the results of high-throughput experiments on gene expression using hybridization-based (e.g., microarray) and synthesis-based (e.g., RNA sequencing) methods2. For my analysis, I specifically looked at differential gene expression in cells from the JJN-3 cell line that either received no treatment or were exposed to 0.4 mM of Amiloride.
The first analysis I did on this data was to create a scatterplot of gene expression for all of the experimental and control groups, as demonstrated in the charts below:
Want to learn data visualization? Check out the featured article below!
I compared the raw gene expression data between the control and treatment groups in the leftmost chart above. In the rightmost chart, I graphed the logarithm (base-10) of the differential gene expression data, which is a common practice in bioinformatics for normalizing data and making it more easily interpreted. From a quick visual inspection, gene expression between the control and treatment groups appears correlated, but certain genes appear to be differentially expressed. To better understand these patterns, I next conducted a t-test to assess whether the mean expression levels of genes are significantly different between our two experimental conditions (e.g., control and treatment) using the code below:
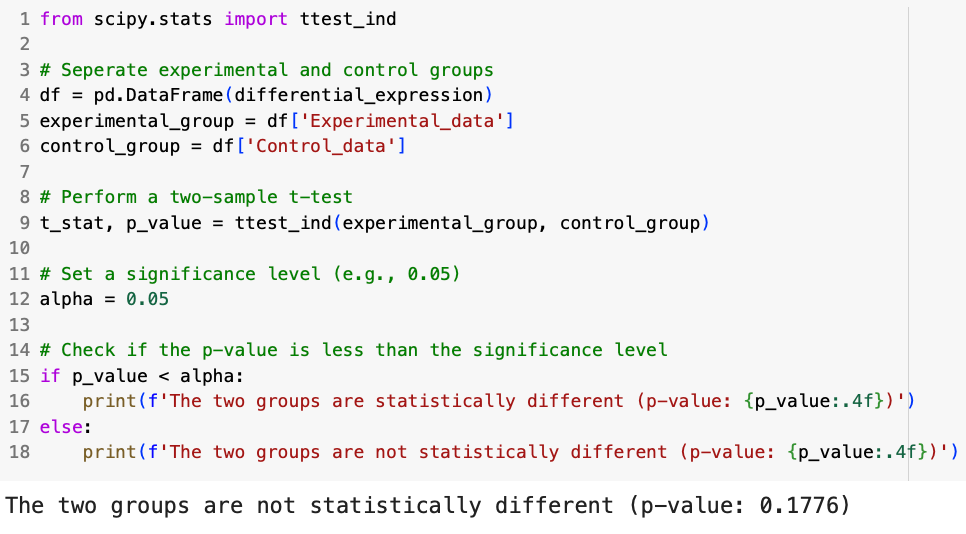
As you can see from the output of my code above, the mean expression levels of genes in our two experimental conditions are not significantly different. However, this does not mean that there aren’t any genes that are not differentially expressed between the control and treatment groups. As a result, the next analysis I did was to view the magnitude of change in gene expression with a mean difference plot, as demonstrated below:
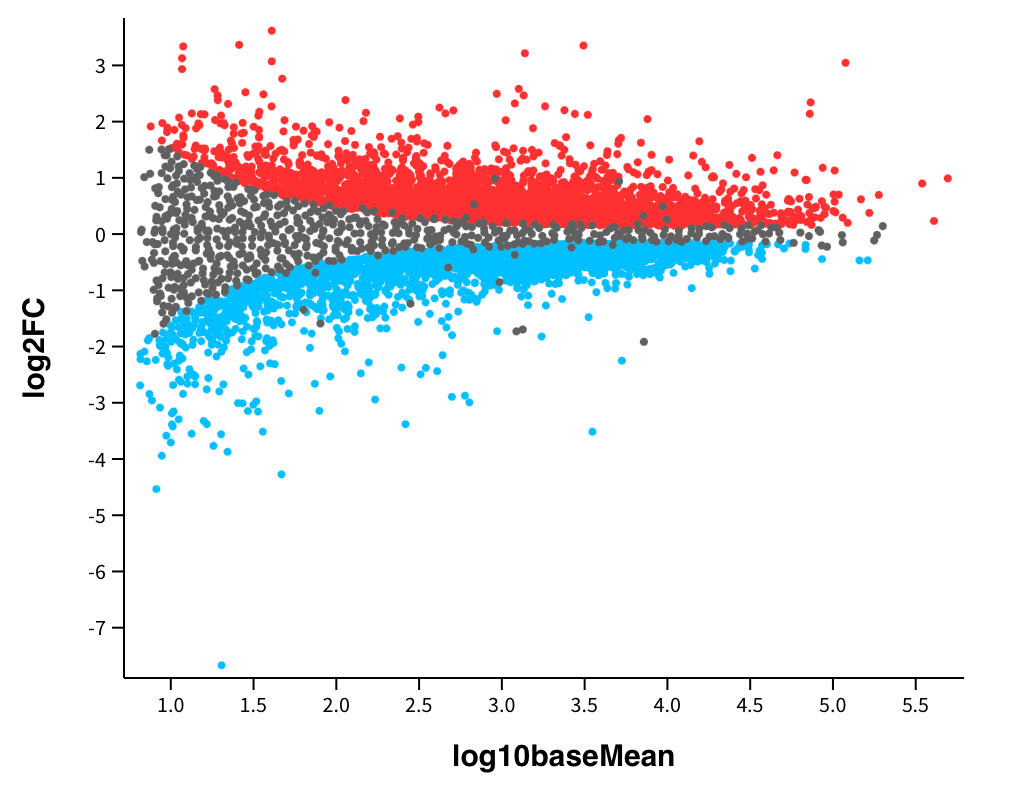
In the image above, the fold change on the y-axis represents the magnitude of the change in gene expression between the control and treatment conditions. The fold change is calculated as the ratio of the expression level in one condition to the expression level in another, and it is used to assess the biological significance of gene expression changes. A gene with a high fold change substantially differs in expression levels between conditions. For example, genes that are highlighted in blue in the image above were downregulated in the treatment group compared to the control group, and genes highlighted in red were unregulated in the treatment group compared to the control group. Additionally, false discovery rate correction was applied to adjust p-values to account for the increased risk of observing statistically significant results by chance when conducting many tests simultaneously. This is essential when conducting thousands of tests in parallel, as is often the case in high-throughput genomics experiments.
After viewing the mean difference plot above, I downloaded a data file from GEO2R with the ~7000 genes that differed most between our two experimental conditions among the ~24,000 genes that were compared. The image below shows a series of box plots comparing gene expression in our treatment and control groups for four of the genes with the greatest differential expression differences from the aforementioned dataset:
Recall that both the control and experimental (Amiloride treatment) groups use the JJN3 cell line, which was established from the bone marrow of a 57-year-old woman with plasma cell leukemia. Thus, we should expect the genes that are upregulated in the control group, compared to the treatment group, to have oncogenic effects. Let’s check them out one by one:
EEF1A (eukaryotic translation elongation factor) is highly expressed in human tumors, including breast, ovarian, and lung cancer3. Based on our analysis we can see that Amiloride treatment reduces EEF1A expression in JJN3 cells by 1.9x, which is statistically significant (p=0.0001).
ALDOA (muscle fructose-1,6-bisphosphate aldolase) has been identified as an oncogene in a variety of tumors and has been reported to facilitate cancer cell proliferation by accelerating glycolysis (ALDOA is also among the most abundant glycolytic enzymes in all cancer cells)456. Our analysis shows that Amiloride treatment reduces ALDOA expression in JJN3 cells by 1.9x, which is statistically significant (p=0.0002).
PH4B (prolyl 4-hydroxylase subunit beta) is a gene that is often highly expressed in various cancer types, including renal, prostate, and ovarian cancer. Additionally, P4HB often increases with cancer grade and is associated with reduced survival, and as a result, it has significant prognostic value78. Based on our analysis, Amiloride treatment reduced PH4B expression by 1.8x, which is statistically significant (p=0.0034).
CD74 is associated with tumor progression and metastasis, and its upregulation has been asserted with poor prognosis and high tumor-infiltrating leucocyte in breast cancer910. Our analysis shows Amiloride treatment reduced CD74 expression by 1.4x, which is statistically significant (p=0.0171).
Based on the limited analysis above, we can see that Amiloride significantly reduces the expression of several cancer-promoting genes. This data recapitulates the results of a handful of preclinical studies exploring the potential anticancer effects of Amiloride. However, Amiloride is not typically prescribed as a primary treatment for cancer. The reason for this lies in our data. Recall the following chart presented earlier in this article:
The figure above shows that Amiloride treatment results in the upregulation and downregulation of several genes. In the analysis above, I specifically mentioned four oncogenic genes whose expression is downregulated following Amiloride treatment. However, there are also multiple oncogenes whose upregulation is increased following Amilioride treatment, such as ACTG1, suggesting that Amilioride may have some cancer-promoting effects.
The relationship between Amiloride and cancer is complex, and the context matters. In certain situations, Amiloride can inhibit ion channels involved in cancer cell proliferation and migration, highlighting its anticancer potential. In other situations, Amiloride may promote cancer progression by influencing tumor microenvironments. By performing differential gene expression analysis, we better understand how a given therapeutic, such as Amiloride, impacts the human body, leading to greater treatment specificity in time.
🧫 Want To Learn More? Check Out The Following Related Newsletters!
Rojas EA, Corchete LA, San-Segundo L, Martínez-Blanch JF, Codoñer FM, Paíno T, Puig N, García-Sanz R, Mateos MV, Ocio EM, Misiewicz-Krzeminska I, Gutiérrez NC. Amiloride, An Old Diuretic Drug, Is a Potential Therapeutic Agent for Multiple Myeloma. Clin Cancer Res. 2017 Nov 1;23(21):6602-6615. doi: 10.1158/1078-0432.CCR-17-0678. Epub 2017 Aug 8. PMID: 28790111.
https://www.ncbi.nlm.nih.gov/geo/query/acc.cgi?acc=GSE95077
Abbas W, Kumar A, Herbein G. The eEF1A Proteins: At the Crossroads of Oncogenesis, Apoptosis, and Viral Infections. Front Oncol. 2015 Apr 7;5:75. doi: 10.3389/fonc.2015.00075. PMID: 25905039; PMCID: PMC4387925.
Gizak A, Wiśniewski J, Heron P, Mamczur P, Sygusch J, Rakus D. Targeting a moonlighting function of aldolase induces apoptosis in cancer cells. Cell Death Dis. 2019 Sep 26;10(10):712. doi: 10.1038/s41419-019-1968-4. PMID: 31558701; PMCID: PMC6763475.
Tian W, Zhou J, Chen M, Qiu L, Li Y, Zhang W, Guo R, Lei N, Chang L. Bioinformatics analysis of the role of aldolase A in tumor prognosis and immunity. Sci Rep. 2022 Jul 8;12(1):11632. doi: 10.1038/s41598-022-15866-4. PMID: 35804089; PMCID: PMC9270404.
Song J, Li H, Liu Y, Li X, Shi Q, Lei QY, Hu W, Huang S, Chen Z, He X. Aldolase A Accelerates Cancer Progression by Modulating mRNA Translation and Protein Biosynthesis via Noncanonical Mechanisms. Adv Sci (Weinh). 2023 Sep;10(26):e2302425. doi: 10.1002/advs.202302425. Epub 2023 Jul 11. PMID: 37431681; PMCID: PMC10502857.
Wang X, Bai Y, Zhang F, Yang Y, Feng D, Li A, Yang Z, Li D, Tang Y, Wei X, Wei W, Han P. Targeted Inhibition of P4HB Promotes Cell Sensitivity to Gemcitabine in Urothelial Carcinoma of the Bladder. Onco Targets Ther. 2020 Sep 28;13:9543-9558. doi: 10.2147/OTT.S267734. PMID: 33061438; PMCID: PMC7532080.
Lyu L, Xiang W, Zheng F, Huang T, Feng Y, Yuan J, Zhang C. Significant Prognostic Value of the Autophagy-Related Gene P4HB in Bladder Urothelial Carcinoma. Front Oncol. 2020 Aug 13;10:1613. doi: 10.3389/fonc.2020.01613. PMID: 32903592; PMCID: PMC7438560.
Gil-Yarom N, Radomir L, Sever L, Kramer MP, Lewinsky H, Bornstein C, Blecher-Gonen R, Barnett-Itzhaki Z, Mirkin V, Friedlander G, Shvidel L, Herishanu Y, Lolis EJ, Becker-Herman S, Amit I, Shachar I. CD74 is a novel transcription regulator. Proc Natl Acad Sci U S A. 2017 Jan 17;114(3):562-567. doi: 10.1073/pnas.1612195114. Epub 2016 Dec 28. PMID: 28031488; PMCID: PMC5255621.
Xu S, Li X, Tang L, Liu Z, Yang K, Cheng Q. CD74 Correlated With Malignancies and Immune Microenvironment in Gliomas. Front Mol Biosci. 2021 Sep 1;8:706949. doi: 10.3389/fmolb.2021.706949. PMID: 34540893; PMCID: PMC8440887.
Here is a thought , history repeats itself ....
I truly believe that this topic should be banned and should have one of the most strict consequences like murder. It should be outlawed and deemed to be taboo , people should be afraid to even talk about the topic .
The arrogance and inability to recognize that they are trying to play God will only end with very bad results. After all humanity was already purged off the face of the earth for going down this very path .
You really think you or anyone else on earth has even the slightest clue to what they are doing with genetics . You need to stop for a minute and step back into reality, take a breath of fresh air and absorb your surroundings. Look at the complexity of everything how it all works and functions . You think you have what it takes to create life ?? Well you don't, naturally yes but playing with genetics hell no. You are going to make a mess and bring about the extinction of mankind ..
Something to think about ...